|
|
Our Technology
|
WAY-2C technology is concerned with identification based on color, false color, spectral
and similar images and/or data sets.
Built on a strong foundation of probability and information theory,
our software is the result of over two decades of intensive R&D by a team of experienced PhDs.
It has been successfully used in a wide variety of commercial applications.
The technology has two distinct aspects, color-based
identification, and optimum subset selection from large
spectral data sets to perform such identification.
As the need for image based identification moves out of
well-controlled stable environments into the world of ever-changing conditions,
traditional machine vision technology has often proven inadequate for the job.
The basic WAY-2C software is a unique system for efficient robust
color-based identification where the colors and shape of the
items of interest and their surroundings may be arbitrarily complex,
and/or change quickly. Unlike traditional methods, it uses very rapid machine learning to routinely
produce far more accurate identification than today's widely taught,
but outdated, color machine vision methods.
Unlike neural network based methods, WAY-2C does not need to be fully
retrained every time an
item in the identification set is added, removed, or otherwise changed.
Items in the set may be easily relearned, added, removed, and/or temporarily
disabled even during production runs.
In spite of its power,
WAY-2C-based applications usually require no special operator skills
for rapid, efficient training and reliable operation.
|
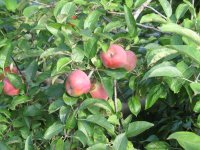
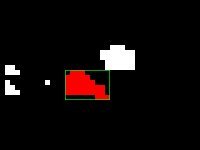
|
|
Multispectral and hyperspectral imaging systems are characterized by the ability
to capture very large amounts of data for analysis.
Often, when the purpose is identification,
this data can be orders of magnitude more than is necessary
to reliably differentiate the specific classes of interest.
Our new method, which we refer to as "Relevance Spectroscopy",
described in detail in US Patent 8,918,347, addresses this problem
in a straighforward, deterministic manner. Once the classes of interest
and their training regions have been specified,
the method searches the entire data set to find an optimum subset
to differentiate the classes.
For typical spectral images,
the resulting subset comes from three or fewer spectral bands,
is one or more orders of magnitude smaller
than the original data set, and determination requires a couple of minutes on a modest PC.
Color images constructed from the subset are ready for any manual or machine interpretation.
|
For more information
 
Applications   
FAQ   
Home   
Index   
Examples   
Info   
Free Trial   
Sales   
Top
 
Last Updated 5/18/18
|